43 min to read
Enter the world of Generative AI
Co-Creating Tomorrow Where Human Ingenuity Meets AI Brilliance.
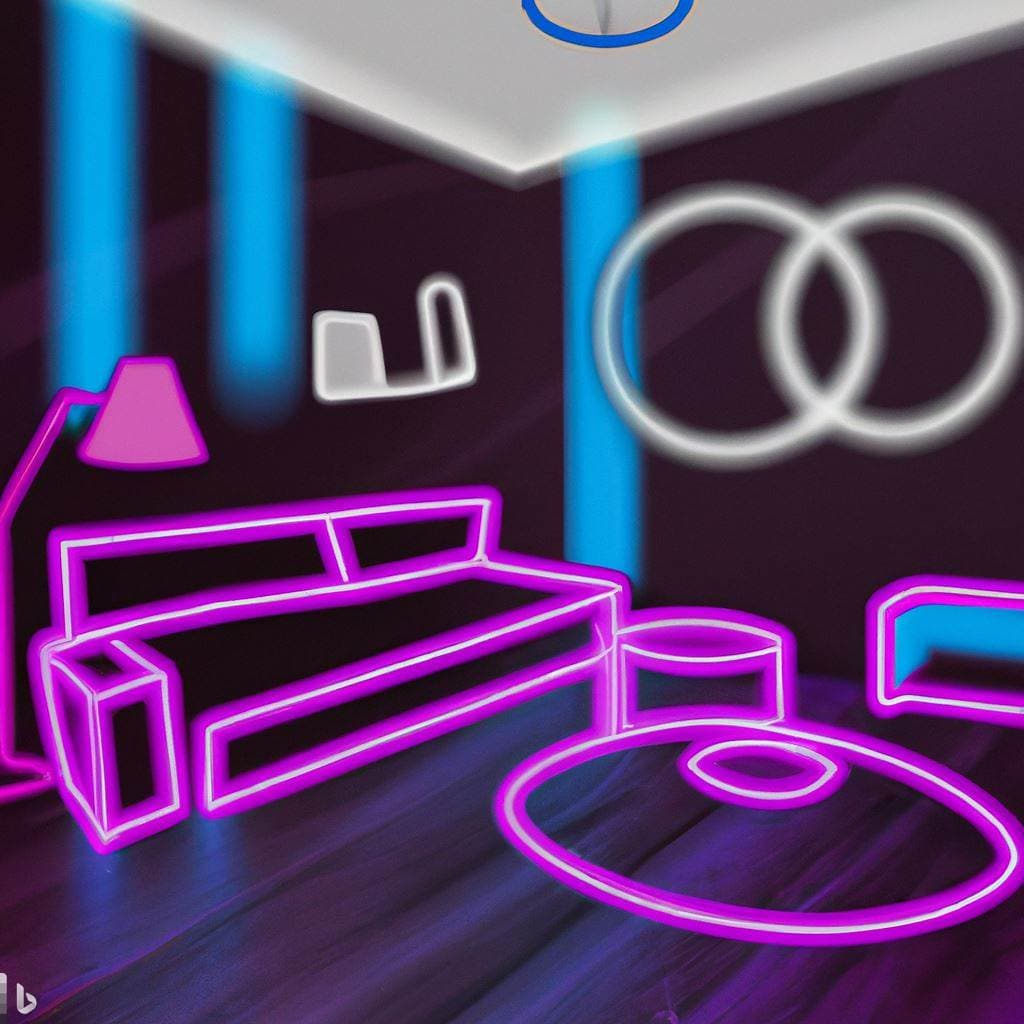
“People worry that computers will get too smart and take over the world, but the real problem is that they’re too stupid and they’ve already taken over the world.” ― Pedro Domingos
Artificial Intelligence (AI) has been a buzzword for the past few years, and it has been transforming the way we live and work. AI has been used in various fields, including healthcare, finance, and entertainment. One of the most exciting areas of AI is Generative AI. This article will explore Generative AI, its applications, and how it works.
Imagine having a digital artist at your fingertips, capable of creating paintings, writing stories, or even composing music. Generative AI is a cutting-edge technology that brings this imagination to life. At its core, it’s a system trained on vast amounts of data, learning patterns, styles, and structures from what it sees. Once trained, it can generate new, original content that resembles what it has learned. Think of it as teaching a child to draw by showing them thousands of pictures. Over time, the child begins to create their own drawings, inspired by what they’ve seen. Generative AI works similarly, offering endless possibilities for creativity and innovation. It’s not just about replicating what exists; it’s about inspiring new ideas and perspectives.
What is Generative AI?
Generative AI is a type of AI that can create new data that is similar to the data it has been trained on. It is a subset of machine learning that uses deep neural networks to generate new data. Generative AI can create images, videos, music, and even text. It is a powerful tool in various fields, including art, design, and entertainment.
How does Generative AI work?
Generative AI uses deep neural networks to learn the patterns in the data it has been trained on. The neural network consists of layers of nodes connected to each other. Each node in the network performs a simple mathematical operation on the input data and passes the result to the next layer. The output of the last layer is the generated data.
The neural network is trained on a dataset containing examples of the data it needs to generate. For example, if we want to generate images of cats, we would train the neural network on a dataset of cat images. During training, the neural network learns the patterns in the data and uses them to generate new data similar to the training data.
Applications of Generative AI
Generative AI has many applications in various fields. Some of the most exciting applications of Generative AI are:
- Art and Design: Generative AI has emerged as a groundbreaking tool in the realms of art and design, blurring the lines between human creativity and machine-generated content. Artists and designers are harnessing the power of AI to create intricate visual masterpieces, from paintings to digital graphics, that are often indistinguishable from human-made works. These algorithms can generate unique patterns, textures, and color palettes, providing artists with a vast canvas of possibilities to augment their creations. In the world of fashion design, AI can predict upcoming trends by analyzing vast datasets, and even suggest novel clothing designs, merging traditional aesthetics with avant-garde styles. Digital sculptors and architects are using generative models to conceive structures and spaces that optimize functionality while maintaining aesthetic appeal. Moreover, interactive art installations now employ AI to evolve in real-time based on audience interactions, offering a dynamic and immersive experience. As the boundaries of what’s possible in art and design continue to expand, Generative AI stands at the forefront, championing a new era of limitless creative potential.
- Gaming: The gaming industry, always at the cutting edge of technological innovation, has embraced Generative AI to elevate the gaming experience to unprecedented levels. One of the most notable applications is in the realm of game design, where AI algorithms can autonomously generate intricate game levels, terrains, and worlds, ensuring each gameplay experience is unique and unpredictable. Character design, too, has seen a revolution, with AI crafting diverse and lifelike NPCs (non-player characters) that react dynamically to player actions, making in-game interactions more immersive and realistic. Beyond design, generative models are being employed to create adaptive soundtracks that change based on gameplay, ensuring the audio environment always complements the on-screen action. Additionally, AI-driven narratives are emerging, where the storyline evolves based on player choices, leading to multiple branching paths and endings. This not only enhances replayability but also offers a deeply personalized gaming experience. As Generative AI continues to evolve, its integration into the gaming industry promises to redefine the boundaries of interactive entertainment.
- Healthcare: In the healthcare industry, Generative AI is becoming a pivotal force, driving advancements that promise better patient outcomes and more efficient medical processes. One of its most transformative applications lies in drug discovery, where AI models can predict the potential efficacy and safety of new compounds, significantly accelerating the traditionally lengthy and costly research phases. Radiology, too, has benefited, with AI algorithms generating detailed and enhanced medical images, aiding in the early detection and diagnosis of diseases. Personalized medicine is another frontier, where generative models can tailor treatments based on an individual’s genetic makeup, ensuring optimal therapeutic results. Additionally, AI-driven simulations can predict the progression of diseases in patients, enabling timely interventions and better care planning. In medical training, generative algorithms are being used to create realistic virtual patients for simulation-based learning, providing medical professionals with hands-on experience without any risk. As the healthcare industry grapples with increasing challenges, from aging populations to emerging diseases, the applications of Generative AI offer hope for more effective, personalized, and timely medical solutions.
- Banking, Financial Services, and Insurance (BFSI): Generative AI is rapidly reshaping the Banking, Financial Services, and Insurance (BFSI) industry, introducing efficiencies and capabilities that were previously unattainable. In banking, AI-driven algorithms can generate predictive models for credit scoring, offering more accurate assessments of loan applicants by analyzing vast datasets beyond traditional metrics. For financial services, generative models assist in algorithmic trading by forecasting market movements, optimizing investment strategies, and simulating various economic scenarios to test the resilience of financial portfolios. In the insurance sector, AI can automate claim processing by generating damage assessments from images or videos of incidents, ensuring faster and more accurate claim settlements. Furthermore, generative AI aids in fraud detection by creating models that can identify unusual transaction patterns, safeguarding both institutions and their customers. As the BFSI industry grapples with increasing data volumes and the need for real-time decision-making, the applications of Generative AI stand as a beacon for innovation, security, and enhanced customer experience.
- Agriculture: Generative AI has the potential to revolutionize the agricultural industry. By leveraging AI-driven technologies, farmers can gain insights into their crops and soil conditions, allowing them to make more informed decisions about managing their land best. Generative AI can also be used to predict weather patterns and optimize irrigation systems, helping farmers maximize crop yields. Additionally, generative AI can identify pests and diseases in crops, enabling farmers to take preventive measures before they become a problem. Finally, generative AI can be used to analyze data from sensors and drones in order to monitor crop health and detect problems early on. All of these applications of generative AI have the potential to improve agricultural productivity and efficiency significantly.
- Education: Generative AI is ushering in a transformative era in the education sector, offering tools and solutions that personalize and enhance the learning experience. One of its standout applications is in content creation, where AI can generate customized learning materials tailored to individual student needs, ensuring that content is both engaging and at the right difficulty level. For educators, generative models can assist in crafting lesson plans, quizzes, and assignments, reducing preparation time and ensuring alignment with curriculum standards. In language learning, AI-driven platforms can produce diverse conversational scenarios, aiding students in mastering linguistic nuances and real-world interactions. Furthermore, virtual labs powered by AI allow students to conduct experiments and simulations in a risk-free environment, fostering hands-on learning and exploration. Additionally, predictive models can forecast students’ academic trajectories, identifying potential areas of struggle and allowing for timely interventions. As education continues to evolve in the digital age, Generative AI stands as a beacon for innovation, ensuring that learning is dynamic, personalized, and accessible to all.
- Media and Communications: Generative AI has revolutionized the landscape of media and communications, offering a plethora of innovative applications that were once deemed futuristic. In the realm of content creation, generative models can autonomously produce written articles, music compositions, and even realistic video footage, reducing the time and effort traditionally required in these processes. For instance, news agencies have started using AI to generate reports on financial data or sports events, ensuring rapid dissemination of information. In film and entertainment, AI-driven tools can create lifelike visual effects, character animations, or even entire movie scenes, pushing the boundaries of what’s possible in storytelling. Furthermore, in personalized advertising, generative AI can tailor promotional content to individual preferences, ensuring higher engagement rates. This technology also aids in real-time language translation and content localization, bridging communication gaps in an increasingly globalized world. As these applications continue to mature, the convergence of generative AI and media promises to redefine the way we create, consume, and communicate content.
- Entertainment Industry: The entertainment Industry has been profoundly transformed by the advent of Generative AI, ushering in a new era of creativity and innovation. In the world of music, AI algorithms can now compose original scores, assist artists in songwriting, and even generate entirely new genres, blending traditional sounds with futuristic beats. In film, generative models are being employed to create hyper-realistic visual effects, simulate crowd scenes, and even design unique characters, reducing the need for extensive manual labor and large production budgets. Video game developers leverage AI to craft expansive, dynamic worlds, where the environment and characters evolve based on player interactions, offering a more immersive gaming experience. Additionally, virtual influencers, entirely crafted by AI, are emerging as new-age celebrities on social media platforms, captivating audiences with their digital personas. As Generative AI continues to evolve, its integration into the entertainment sector promises to deliver experiences that are not only entertaining but also unprecedented in their depth and realism.
Generative AI’s capability to produce synthetic data has become a game-changer for numerous industries and domains, addressing challenges related to data scarcity, privacy, and quality. In sectors where real data is limited or expensive to obtain, such as rare medical conditions or niche market research, AI can generate representative datasets, enabling robust analysis and model training. For industries concerned with privacy, like healthcare or finance, generative models can create synthetic datasets that mimic the statistical properties of the original data without containing any personally identifiable information, ensuring compliance with data protection regulations. In domains where data quality and diversity are paramount, such as autonomous vehicle development or AI model validation, synthetic data can augment existing datasets, introducing scenarios or edge cases that might be rare in real-world data but are crucial for comprehensive testing. By bridging data gaps, enhancing quality, and ensuring privacy, Generative AI’s prowess in synthetic data generation is paving the way for more robust, accurate, and ethical applications across diverse fields.
Generative AI’s capacity to produce synthetic data offers a transformative solution to many industries and domains, addressing challenges tied to data limitations, privacy concerns, and the need for diverse datasets. Here’s a deeper dive with examples:
Healthcare: Real patient data is sensitive, and sharing it can violate privacy regulations. However, research often requires vast amounts of data. Generative AI can create synthetic patient records that maintain the statistical properties of real data without compromising individual identities. For instance, a research institution studying a rare disease might not have access to a large number of patient records. Using Generative AI, they can amplify their dataset with synthetic records, enabling more comprehensive research.
Finance: Financial institutions need to test their systems against various economic scenarios, some of which might be rare or unprecedented. Instead of waiting for real-world data, Generative AI can simulate financial market conditions, helping institutions prepare for diverse economic events. For example, a bank could use synthetic data to model the impact of a sudden, large-scale market crash, ensuring their systems and strategies are robust against such events.
Autonomous Vehicles: Training autonomous vehicles requires vast amounts of driving data, especially for rare but critical events like a child running onto the road. Generative AI can create synthetic scenarios that might be infrequent in real-world driving datasets but are essential for comprehensive training. This ensures that the vehicle’s AI is well-prepared for a wide range of on-road situations.
Retail and E-commerce: Companies often want to understand consumer behavior in new markets or under hypothetical promotional scenarios. Instead of real-world trials, Generative AI can simulate customer purchasing behaviors based on existing data, helping businesses strategize effectively. For instance, an e-commerce platform can generate synthetic data to predict how consumers might react to a new pricing strategy during a holiday season.
Energy: For sectors like renewable energy, predicting equipment failures or energy yields under various conditions is crucial. Generative AI can simulate weather patterns or equipment wear-and-tear scenarios, allowing energy providers to optimize maintenance schedules and energy distribution strategies.
In essence, Generative AI’s ability to produce synthetic data not only fills data gaps but also allows industries to model, predict, and prepare for a myriad of scenarios, ensuring robustness and preparedness in their respective domains.
Creating synthetic data involves generating data that mimics the properties and patterns of real data without directly copying it. This is often achieved using various statistical and machine learning methods. Here’s a step-by-step process to create synthetic data:
Define the Objective: Understand why you need synthetic data. Is it for data augmentation, privacy preservation, or simulating rare events? Your objective will guide the subsequent steps.
Collect and Analyze Real Data: Before generating synthetic data, you need a real dataset to serve as a reference. Analyze this dataset to understand its structure, patterns, and statistical properties.
Choose a Method:
- Statistical Methods: For simpler datasets, statistical methods like bootstrapping (resampling with replacement) or generating data from known distributions (e.g., Gaussian) might suffice.
- Machine Learning Models: For complex datasets, models like Variational Autoencoders (VAEs) or Generative Adversarial Networks (GANs) are popular choices. These models can capture intricate patterns and relationships in the data.
Data Preprocessing: Clean and preprocess the real data. This might involve normalization, handling missing values, and encoding categorical variables.
Train the Model (if using ML methods):
- For GANs: Train both the generator (creates synthetic data) and the discriminator (distinguishes between real and synthetic data) in tandem. The generator improves iteratively based on feedback from the discriminator.
- For VAEs: Train the model to encode data into a lower-dimensional space and then decode it back. The encoding captures the essential features of the data.
Generate Synthetic Data:
- Statistical Methods: Sample from the distributions or resample the real data.
- Machine Learning Models: Use the trained generator (in GANs) or the decoder part of VAEs to produce synthetic data.
Post-process Synthetic Data: This might involve reversing any normalization or encoding done during preprocessing.
Evaluate Quality and Privacy:
- Quality: Compare the statistical properties (e.g., mean, variance) of the synthetic data to the real data. Also, check if the synthetic data preserves relationships and patterns from the real data.
- Privacy: Ensure that the synthetic data doesn’t contain information that can be traced back to individual records in the real dataset. Differential privacy techniques can be applied to add noise and further ensure privacy.
Iterate: Based on the evaluation, you might need to adjust your methods or model parameters and regenerate synthetic data.
Use Synthetic Data: Once satisfied, use the synthetic data for your intended purpose, whether it’s model training, testing, or analysis.
Remember, while synthetic data can be immensely valuable, it’s essential to ensure that it’s of high quality and serves the intended purpose without introducing biases or inaccuracies.
It’s not just about creating data but also about crafting experiences, solutions, and insights across diverse domains.
Crafting Experiences:
- Interactive Media and Gaming: Generative AI can create dynamic game environments that respond to a player’s actions, offering a unique experience each time the game is played. For instance, terrain, weather, or even storyline elements can be generated on-the-fly based on player choices.
- Virtual Reality (VR) and Augmented Reality (AR): In VR/AR, generative models can craft immersive environments or scenarios, enhancing user immersion. For example, a VR training program for firefighters might use AI to generate unpredictable fire patterns, ensuring trainees experience a wide range of scenarios.
- Personalized Content: Generative AI can tailor content to individual preferences, whether it’s a music playlist, a news feed, or a shopping recommendation, enhancing user engagement and satisfaction.
Crafting Solutions:
- Drug Discovery: Generative models can propose potential molecular structures for new drugs, speeding up the initial phases of drug development.
- Design and Architecture: AI can assist designers by generating preliminary design concepts based on specified criteria, streamlining the creative process.
- Optimization Problems: In logistics, transportation, or manufacturing, generative models can simulate various scenarios to find optimal solutions, such as the best route for delivery or the most efficient production schedule.
Crafting Insights:
- Predictive Analytics: By simulating potential future scenarios, businesses can gain insights into market trends, customer behavior, or potential risks. For instance, a retailer might use generative AI to predict sales during a promotional event.
- Anomaly Detection: In sectors like finance or cybersecurity, generative models can learn the patterns of normal transactions or network traffic. Once trained, they can identify anomalies or suspicious activities, providing insights into potential fraud or security breaches.
- Research and Development: In fields like climate science, astrophysics, or economics, generative models can simulate complex systems, offering researchers insights into phenomena that are difficult or impossible to observe directly.
In essence, Generative AI’s ability to craft experiences, solutions, and insights means it’s not just a tool for creating data but a comprehensive solution that can enhance user experiences, solve complex problems, and provide valuable insights across a wide range of industries and domains.
Exploring and working in the field of Generative AI requires a combination of foundational knowledge, technical skills, and an understanding of the ethical and practical implications of the technology. Here’s a comprehensive list of what one might need:
Foundational Knowledge:
- Machine Learning Basics: Understand supervised, unsupervised, and reinforcement learning paradigms.
- Deep Learning: Grasp the concepts of neural networks, backpropagation, activation functions, and architectures like CNNs (Convolutional Neural Networks) and RNNs (Recurrent Neural Networks).
- Probability and Statistics: Generative AI often involves probabilistic models, so a solid grounding in statistics is crucial.
Technical Skills:
- Programming: Proficiency in languages like Python, which is widely used in AI research and applications.
- Frameworks: Familiarity with deep learning frameworks like TensorFlow, PyTorch, and Keras.
- Model Architectures: Deep dive into specific generative models like GANs, VAEs, and others.
- Optimization: Understand optimization techniques and algorithms, as they play a crucial role in training generative models.
Domain-Specific Knowledge:
- Depending on where you apply Generative AI, domain expertise can be invaluable. For instance, applying Generative AI in healthcare might require some understanding of biology or medicine.
Ethical Considerations:
- Bias and Fairness: Recognize that AI models can inherit biases from training data and understand methods to mitigate these biases.
- Privacy: Learn about techniques like differential privacy that help in generating data without compromising individual privacy.
- Authenticity: Understand the implications of generating realistic fake content, especially in areas like deepfakes.
Practical Skills:
- Data Handling: Know how to preprocess, clean, and manage large datasets.
- Computational Skills: Familiarity with GPU computing, parallel processing, and cloud platforms like AWS, Google Cloud, or Azure.
- Model Evaluation: Ability to evaluate the performance and authenticity of generative models.
Continuous Learning:
- Research Acumen: The field of Generative AI is rapidly evolving. Regularly read research papers, attend conferences, and engage with the AI community.
- Experimentation: Practical hands-on experience is invaluable. Regularly experiment with different models and datasets.
Soft Skills:
- Problem-Solving: Ability to approach challenges methodically and find innovative solutions.
- Collaboration: Often, AI projects are interdisciplinary, requiring collaboration with domain experts, data engineers, and other stakeholders.
- Communication: Ability to explain complex AI concepts to non-experts, especially when discussing the potential and limitations of generative models.
Networking:
- Engage with the AI community through forums, workshops, online platforms like GitHub, and organizations like OpenAI or DeepMind.
Entering the field of Generative AI is undoubtedly challenging, given its interdisciplinary nature and rapid advancements. However, with dedication, continuous learning, and hands-on experience, one can become proficient and contribute meaningfully to this exciting domain.
The principles and techniques of data science provide the groundwork upon which many AI models, including generative ones, are built.
Data Collection and Preprocessing:
- Data Acquisition: Understanding where and how to gather relevant data is crucial. This might involve web scraping, accessing APIs, or using specialized sensors.
- Data Cleaning: Raw data often contains errors, missing values, or inconsistencies. Data science techniques help in cleaning and structuring this data for model training.
- Feature Engineering: Transforming or combining raw data attributes to create more informative features can significantly impact the performance of generative models.
Exploratory Data Analysis (EDA):
- Before diving into complex generative models, it’s essential to understand the underlying patterns, correlations, and distributions in the data. EDA techniques, including visualization, provide insights into these aspects.
Statistical Foundations:
- Generative AI models, especially those like Variational Autoencoders, have a strong grounding in probability and statistics. Knowledge of distributions, statistical testing, and estimation techniques is vital.
Dimensionality Reduction:
- Techniques like PCA (Principal Component Analysis) or t-SNE can be useful, especially when dealing with high-dimensional data. They can help in visualizing data clusters or simplifying data before feeding it into generative models.
Model Validation:
- Understanding techniques like cross-validation ensures that generative models are evaluated rigorously, reducing the risk of overfitting.
Optimization:
- Many generative models involve optimization problems, where the goal is to find the best parameters that minimize (or maximize) a particular function. Knowledge of optimization algorithms and techniques is crucial.
Scalability and Big Data:
- Generative AI models often require large datasets for training. Familiarity with big data tools like Hadoop or Spark and concepts like distributed computing can be beneficial when handling vast amounts of data.
Interpretability and Explainability:
- While generative models can produce impressive results, understanding why and how they work is equally important. Data science offers tools and techniques to interpret and explain model outcomes.
Ethical and Responsible AI:
- Data science emphasizes the responsible use of data, considering aspects like bias, fairness, and privacy. These principles are equally essential when working with Generative AI.
In essence, data science provides the foundational tools and methodologies upon which Generative AI is built. A strong grasp of data science concepts ensures that one can effectively harness the power of generative models, from data collection to model deployment.
Examples of Generative AI
Generative AI has made significant strides in various domains, leading to applications that were once considered science fiction. Here are some notable examples:
Deepfakes: Deepfakes involve generating realistic-looking video footage of real people saying or doing things they never did. This is achieved by training a model on numerous images and videos of the target person. While there are creative uses, such as in movies or entertainment, deepfakes also pose ethical concerns, especially when used for misinformation or defamation.
DeepDream: DeepDream is a Generative AI model that can generate surreal and dream-like images. Google developed it, and it has been used in various applications, including art and design.
Art Creation: Generative AI can produce paintings, drawings, or other visual art forms. Platforms like Artbreeder allow users to blend and modify images using generative models. Additionally, AI-generated art pieces have been auctioned at renowned places like Christie’s.
Music Composition: AI models can compose original music pieces in various styles and genres. OpenAI’s MuseNet is an example that can generate compositions in styles ranging from classical to contemporary.
Text Generation: Advanced models can produce coherent and contextually relevant paragraphs of text. OpenAI’s GPT series (like GPT-4) can craft essays, answer questions, write poetry, and even generate code based on prompts.
- GPT-3: GPT-3 is a Generative AI model that can generate human-like text. OpenAI developed it and has been used in various applications, including chatbots and language translation.
- GPT-4: Generative Pre-trained Transformer 4 is a state-of-the-art deep learning model for natural language understanding and generation. Developed by OpenAI, it builds upon the previous iterations of the GPT series, incorporating even more parameters and training data. The model is trained on diverse internet text, allowing it to generate human-like text in response to a given prompt. GPT-4’s architecture consists of transformer layers that enable it to capture complex relationships in language, making it highly versatile in various applications such as text completion, translation, summarization, and question-answering. Its immense scale and capabilities have made it a significant milestone in artificial intelligence, pushing the boundaries of what machines can understand and create in terms of language.
Image Synthesis: AI can generate entirely new images or modify existing ones. NVIDIA’s StyleGAN is known for generating hyper-realistic, yet entirely synthetic, human faces. Another example is DALL·E from OpenAI, which creates unique images from textual descriptions.
- StyleGAN: StyleGAN is a Generative AI model that can generate high-quality images of faces. NVIDIA developed it, and it has been used in various applications, including art and design.
Drug Discovery: Generative models can propose molecular structures for potential new drugs. Atomwise uses AI for drug discovery, predicting which molecules might have therapeutic properties for specific diseases.
Fashion and Design: AI can suggest new clothing designs or patterns. Platforms like Stitch Fix use AI to assist in fashion design, tailoring styles to individual user preferences.
Video Game Environments: Generative AI can craft dynamic game levels or environments. Games like “No Man’s Sky” use procedural generation (a form of generative AI) to create vast, diverse planetary environments for players to explore.
Personalized Content: AI can tailor content, such as news articles or advertisements, to individual user preferences. News platforms might use generative models to craft summaries or headlines tailored to a user’s reading habits.
3D Model Generation: AI can assist in creating detailed 3D models for various applications. In architecture or product design, AI can suggest optimizations or variations to existing 3D models.
These examples showcase the versatility and potential of Generative AI across different sectors. However, with its capabilities come ethical considerations, especially in areas like deepfakes or personalized content, emphasizing the need for responsible use and regulation.
Related concepts
- GANs are like a continuous game between two players (a forger and a detective) where one is trying to produce fake data and the other is trying to detect it. Through this game, the system learns to produce very realistic data.
- DCGAN is like our original game between the forger and detective, but with upgraded, advanced tools that handle digital images. These tools can dive deep into the details of images, making the game even more challenging and resulting in very realistic generated photos.
- WGAN-GP is like an advanced art competition between our forger and detective. The detective uses a sophisticated scoring system to rate the forger’s work, and rules are in place to ensure the forger genuinely improves their skills. This results in artwork (or in the case of computers, generated data) of very high quality.
- CGAN is like our art competition between the forger and detective, but with an added twist of themed challenges. The forger has to create artwork based on a specific theme, and the detective checks both the authenticity and theme adherence. In the computer world, this allows for more controlled and specific data generation.
- SAGAN is like our art competition between the forger and detective, but with the added advantage of a special magnifying glass that emphasizes important details. This ensures that the forger creates more detailed and refined artwork, and the detective inspects with greater precision. In the realm of computers, this results in generating more detailed and coherent data.
- BigGAN is like a grand art collaboration on a massive canvas. It’s a beefed-up version of GANs, designed to produce very high-quality, detailed, and realistic images by leveraging its large size and capacity. Just as a team of artists can create a more intricate masterpiece, BigGAN aims to generate some of the most impressive and detailed digital creations in the GAN world.
- ProGAN is like constructing a house layer by layer, starting from a basic structure and progressively adding more detail. In the digital world, it means starting with a low-resolution image and gradually increasing its clarity and detail until a high-resolution, realistic image is produced. This progressive approach ensures stability and high quality in the generated images.
- VQ-GAN is like recreating a painting using a box of puzzle pieces. Instead of drawing every detail, you assemble the image using predefined pieces, adjusting and refining as needed. In the digital realm, this approach allows for efficient and high-quality image generation by using a set of predefined vectors to construct the image.
- ViT-VQ-GAN combines the strengths of Vision Transformers and VQ-GAN. It uses ViT to break down images into essential patches and then employs VQ-GAN to reconstruct and enhance these images using a set of predefined vectors. The result is a detailed and coherent image generation. ViT-VQ-GAN is like recreating a story in a library. You use summaries to grasp the main themes and then dive into detailed books to enrich and complete your tale. In the realm of image generation, this approach ensures both efficiency and high-quality results.
- StyleGAN2 is like preparing for a grand fashion show. As a designer, you mix styles from different eras, focus on the details, and ensure that every outfit is perfect. In the digital world, StyleGAN2 combines and fine-tunes styles to produce high-quality, diverse, and realistic images, all while improving upon the flaws of its predecessor. StyleGAN2 addresses and corrects certain visual artifacts and issues found in the original StyleGAN, resulting in more realistic and higher-quality image generation.
Read more on GAN here
- Google machine learning GANs
- How can generative adversarial networks learn real-life distributions easily
- A deep generative model trifecta: Three advances that work towards harnessing large-scale power
Multimodal model
In the realm of artificial intelligence, a multimodal model is like this Swiss Army Knife. Instead of processing just one type of data (like text or images), a multimodal model can handle multiple types of data simultaneously. It can understand text, images, sounds, and even videos, often all at once. They’re versatile tools that can process and understand multiple types of data simultaneously, giving them a richer and more holistic understanding of information.
Let us focus on Text for a while. Text generation using large language models in Generative AI has various applications across various domains. Here’s an overview of some of the key applications:
Content Creation and Writing Assistance:
- Automated Journalism: Generating news articles, reports, and summaries.
- Creative Writing: Assisting in writing novels, scripts, poetry, etc.
- Academic Writing: Assisting researchers in drafting papers, abstracts, and literature reviews.
Language Translation:
- Real-time Translation: Translating text between languages in real-time.
- Literary Translation: Translating literature while preserving stylistic nuances.
Education and Tutoring:
- Personalized Learning: Creating customized learning materials for students.
- Homework Assistance: Providing help with homework and assignments.
Marketing and Advertising:
- Content Marketing: Generating marketing content, such as blog posts, social media updates, etc.
- Personalized Advertising: Creating personalized ad copy for targeted audiences.
Customer Support and Engagement:
- Chatbots: Building conversational agents to handle customer queries.
- Email Automation: Crafting personalized emails for customer engagement.
Healthcare:
- Medical Reporting: Generating medical reports and summaries.
- Mental Health Support: Providing therapeutic conversation through AI-driven chatbots.
Entertainment and Gaming:
- Story Generation: Creating engaging stories for games and entertainment.
- Dialogue Writing: Crafting dialogue for video games and movie characters.
Legal and Compliance:
- Contract Generation: Automating the creation of legal documents and contracts.
- Compliance Reporting: Assisting in generating compliance reports and documentation.
Finance and Economics:
- Financial Reporting: Creating financial summaries and reports.
- Risk Analysis: Generating textual insights for risk assessment.
Research and Development:
- Data Analysis: Summarizing and interpreting complex data sets.
- Scientific Discovery: Assisting in hypothesis generation and experimental design.
Accessibility:
- Text-to-Speech: Converting text into natural-sounding speech for visually impaired users.
- Language Simplification: Rewriting complex texts into simpler forms for readers with different comprehension levels.
Human Resources and Recruitment:
- Resume Screening: Automating the screening of resumes and cover letters.
- Job Description Generation: Creating detailed and tailored job descriptions.
E-commerce:
- Product Description Generation: Writing unique and engaging product descriptions.
- Customer Review Analysis: Summarizing and interpreting customer reviews.
Disaster Response and Management:
- Emergency Alerts: Generating real-time alerts and information during disasters.
- Situation Analysis: Providing textual analysis of ongoing emergency situations.
Language Preservation:
- Documenting Endangered Languages: Assisting in documenting and preserving languages at risk of extinction.
These applications demonstrate the versatility and potential of text generation in various fields, contributing to efficiency, creativity, personalization, and accessibility. The ongoing advancements in Generative AI and large language models continue to expand the horizons of what’s possible with text generation.
Real-time business scenarios that leverage AI are becoming increasingly prevalent across various industries. Many AI platforms and portals offer solutions tailored to specific business needs. Here’s an overview of some common real-time business scenarios and how they are explained or implemented across various AI sites and portals:
Customer Service Chatbots: Providing instant customer support through AI-powered chatbots. AI portals offer chatbot solutions that can handle customer inquiries 24/7, providing immediate responses, routing queries to human agents when necessary, and gathering customer feedback.
Fraud Detection: Identifying and preventing fraudulent activities in real-time. AI platforms provide algorithms that analyze transaction patterns and detect unusual behavior, alerting businesses to potential fraud and taking immediate preventive actions.
Supply Chain Optimization: Managing and optimizing the supply chain in real-time. AI solutions analyze real-time data from suppliers, inventory, and logistics to optimize the supply chain, reduce costs, and enhance efficiency.
Predictive Maintenance: Predicting equipment failure and scheduling maintenance. AI portals offer predictive analytics that monitor machinery and equipment, predicting when maintenance is needed, thereby reducing downtime and maintenance costs.
Real-time Marketing Personalization: Personalizing marketing content and offers based on real-time user behavior. AI platforms analyze user behavior and preferences in real-time to deliver personalized content, advertisements, and product recommendations.
Healthcare Patient Monitoring: Continuous monitoring of patient health and vital signs. AI solutions provide real-time analysis of patient data, alerting healthcare providers to changes in patient conditions and enabling timely interventions.
Traffic Management and Optimization: Managing and optimizing traffic flow in urban areas. AI portals offer solutions that analyze real-time traffic data, adjust traffic signals, and provide routing recommendations to reduce congestion.
Energy Management: Real-time monitoring and optimization of energy consumption. AI platforms provide tools to analyze energy usage patterns, optimize energy consumption, and reduce costs in industrial and commercial settings.
Sentiment Analysis for Social Media Monitoring: Analyzing social media sentiment in real-time. AI solutions monitor social media platforms, analyzing public sentiment towards brands, products, or events, allowing businesses to respond promptly.
Real-time Financial Trading: Automated trading based on real-time market data. AI portals offer algorithms that analyze market trends and execute trades in real time, maximizing profits and minimizing risks.
Human Resource Management: Real-time employee performance and engagement tracking and analysis. AI solutions provide insights into employee behavior, performance, and satisfaction, enabling timely interventions and support.
Retail Inventory Management: Managing retail inventory in real-time. AI platforms analyze sales, returns, and inventory levels, automating restocking and optimizing inventory management.
These scenarios are often showcased on AI portals with case studies, demonstrations, and detailed explanations of the underlying technology. They illustrate how AI can transform traditional business processes, adding value through automation, personalization, and real-time insights. Many AI providers also offer customized solutions to specific industry needs and challenges.
Below are examples of real-time business scenarios implemented by various companies and platforms, along with links to their websites where you can find more information:
Intercom, Customer Service Chatbots: Offers chatbot solutions for customer engagement and support. Intercom
Kount, Fraud Detection: Provides real-time fraud prevention and identity verification. Kount
Llamasoft, Supply Chain Optimization: Offers AI-driven supply chain analytics and insights. Llamasoft
Uptake, Predictive Maintenance: Specializes in industrial AI and IoT for predictive maintenance. Uptake
Dynamic Yield, Real-time Marketing Personalization: Offers AI-powered personalization across web, apps, email, and kiosks. Dynamic Yield
Philips, Healthcare Patient Monitoring: Provides patient monitoring solutions using AI. Philips Healthcare
Siemens Mobility, Traffic Management and Optimization: Offers intelligent traffic systems for urban areas. Siemens Mobility
Schneider Electric, Energy Management: Provides real-time energy management solutions. Schneider Electric
Brandwatch, Sentiment Analysis for Social Media Monitoring: Offers social listening and analytics tools. Brandwatch
AlgoTrader, Real-time Financial Trading: Provides automated algorithmic trading solutions. AlgoTrader
Workday, Human Resource Management: Offers HR management software with real-time analytics. Workday
Luminate, Retail Inventory Management: Provides AI-driven retail and inventory management solutions. Luminate Commerce
These examples represent diverse industries and applications using AI in real-time scenarios. You can explore detailed information, case studies, and demonstrations of how these companies leverage AI to enhance their business processes by visiting these websites.
Let’s explore various AI-driven transformations, including text-to-text, text-to-image, image-to-image, text-to-audio, audio-to-text, text-to-video, text-to-code, and text-to-avatar, along with examples of platforms or tools that provide these capabilities:
Text-to-Text (T2T): Converting or translating text into another form of text, such as summarization, translation, or paraphrasing. Google Translate for language translation. Google Translate
Text-to-Image (T2I): Generating images based on textual descriptions. DeepAI’s Text to Image API creates visual representations of textual input. DeepAI
Image-to-Image (I2I): Transforming one image into another, such as style transfer or image-to-image translation. NVIDIA’s Pix2Pix for image-to-image translation. NVIDIA Research
Text-to-Audio (T2A): Converting text into speech or audio format. Amazon Polly for text-to-speech synthesis. Amazon Polly
Audio-to-Text (A2T): Transcribing audio into written text. Google’s Speech-to-Text for audio transcription. Google Speech-to-Text
Text-to-Video (T2V): Creating videos based on textual descriptions or scripts. Runway’s text-to-video synthesis for generating videos from text. Runway
Text-to-Code (T2C): Generating code snippets or full programs based on textual descriptions. OpenAI’s Codex for translating natural language queries into code. OpenAI Codex
Text-to-Avatar (T2A): Creating or controlling virtual avatars based on text input. Facebook’s Meta Avatars for creating personalized avatars from text descriptions. Meta Avatars, now Meta Quest 2 and Meta Quest for Creators
These transformations represent the cutting edge of AI technology, enabling seamless conversion between different forms of media and information. They have wide-ranging applications across industries, including entertainment, education, healthcare, and marketing. By leveraging these tools, businesses and individuals can create more engaging and personalized content, automate tedious tasks, and enhance accessibility.
Conclusion
Generative AI is a powerful tool that can be used in various fields, including art, design, and entertainment. It uses deep neural networks to learn the patterns in the data it has been trained on and generate new data similar to the training data. Generative AI has many applications, including art and design, gaming, healthcare, and finance. With the development of new Generative AI models, we can expect to see more exciting applications of Generative AI in the future.
“Generative AI can mimic the patterns of human creativity, crafting compositions that resonate with human artistry. Yet, while it navigates the vast oceans of possibility, it sails without a compass of emotion, intuition, and the ineffable spark that makes human creativity a profound exploration of our very soul.”
Generative AI and human creativity - the debate and conversation around AI’s role in creativity are significant and growing..
Generative AI models, such as those used for natural language generation, art creation, or other creative tasks, come with the potential for innovation and challenges that must be addressed responsibly. Here are some of the concerns and evils, along with the principles for responsible AI that could counter them:
Potential Evils of Generative AI:
Misinformation and Fake Content: Generative AI can create realistic but false information that might be used to deceive, manipulate opinions, or spread fake news.
Loss of Jobs: Automating creative tasks that were traditionally human might lead to job displacement in various industries.
Bias and Discrimination: If the training data includes biases, the generative models may perpetuate or even exacerbate these biases, leading to unfair or discriminatory outcomes.
Loss of Human Creativity: Relying heavily on AI for creative tasks might undermine the value of human creativity and intuition.
Ethical Implications in Art and Content Creation: Questions about authenticity, ownership, and copyright can arise when AI generates art or literary works.
Security Concerns: There may be potential use in fraudulent activities, deepfakes, and other malicious applications.
Principles for Responsible AI:
Transparency: Clear communication about how and why AI is used, and the logic behind its decisions.
Fairness: Ensuring AI models are trained on diverse and representative data to prevent biases and make equitable decisions.
Accountability: Implementing oversight and responsibility for the outcomes generated by AI, including a clear path for grievances.
Safety and Security: Implementing robust measures to prevent the malicious use of generative AI, including stringent controls over who has access and how it’s used.
Ethical Considerations: Developing a strong ethical framework that guides the development and deployment of AI, considering human values, rights, and potential societal impacts.
Collaboration and Inclusivity: Encouraging a multi-stakeholder approach that includes not just technologists but also ethicists, social scientists, and representatives from diverse communities affected by the technology.
Sustainability: Ensuring that the development and use of AI align with broader social and environmental goals, such as reducing energy consumption.
At this point, it’s worth gaining an understanding of hallucination. Hallucination is a situation where models like OpenAI’s GPT series generate text that doesn’t accurately reflect reality. This can manifest as generating incorrect information, inventing details, or providing overly confident statements about ambiguous or subjective matters. Such hallucinations are considered artifacts of the training data and the modeling process. They can occur when the model makes connections or generalizations that don’t hold true in a specific context or faces an input dissimilar to what it encountered during training. OpenAI and other organizations are continually working to understand and reduce these kinds of issues, but they remain a known limitation of these models. Please take a look at
- Sources of Hallucination by Large Language Models on Inference Tasks.
- Survey of Hallucination in Natural Language Generation
Image generation
Stable Diffusion -Imagine you’re blending two colors of paint: blue and yellow. When you first start mixing, you’ll have areas that are very blue and areas that are very yellow. As you continue to mix, you’ll get a consistent green color throughout. This even mixing is similar to “stable diffusion.” Think of generative AI as an artist trying to paint a picture. Instead of using brushes and paint, it uses data and algorithms. When the AI is “painting” or generating an image, it blends different features and details together, much like mixing colors. “Stable diffusion” in this context means that the AI smoothly and consistently blends these features. Instead of suddenly having a random patch of color (or an unexpected feature in the image), the AI ensures that all elements in the generated image transition smoothly and make sense together, resulting in a coherent and realistic image. In simpler terms, stable diffusion ensures that the AI-generated image looks natural and seamless without any jarring or out-of-place elements.
In conclusion, while Generative AI offers unprecedented opportunities for innovation and efficiency, it also presents significant challenges and potential harms. A responsible approach to AI requires a thoughtful and holistic consideration of these challenges, guided by principles prioritizing human well-being, fairness, transparency, and societal values.
Further references
- DreamBooth: Fine Tuning Text-to-Image Diffusion Models for Subject-Driven Generation
- High-Resolution Image Synthesis and Semantic Manipulation with Conditional GANs
- Denoising Diffusion Implicit Models
- A walk through latent space with Stable Diffusion
- Stable Diffusion blog on hugging face
- CLIP: clip-retrieval - Contrastive Language-Image Pre-training
- OpenAI Research - CLIP
- https://github.com/openai/CLIP
- Multi-modal ML with OpenAI’s CLIP
- OpenCLIP- OpenAI’s CLIP
- High-Resolution Image Synthesis with Latent Diffusion Models
- Computer vision research
- Lambdalabs
- StyleGAN - Music
- TTS - Audio Neural Dialogue Audiolizer
Text-to-Video
- Meta AI Make-A-Video Studio
- Video Diffusion Models
- Awesome Video Diffusion
- https://huggingface.co/blog/text-to-video
- https://imagen.research.google/video
- https://phenaki.video
- https://huggingface.co/spaces/damo-vilab/modelscope-text-to-video-synthesis
- https://github.com/VideoCrafter/VideoCrafter
- https://github.com/THUDM/CogVideo
- https://github.com/topics/text-to-video
- Stability AI - Introducing Stable Video Diffusion
LLMs Leaderboard
- Alpaca Eval Leaderboard
- Open LLM Leaderboard
- LLM Sys projects
- A list of open LLMs available for commercial use
- Aviary Explorer
Govt of India’s initiative on Generative AI
Suggested courses
- Deep Learning.AI Learn Generative AI Short Courses
- AI for Good Specialization by DeepLearning.AI
- Harvard Course - CS50-Introduction to Programming with Python
- Stanford CS229-Machine Learning by Andrew Ng
- Stanford CS229: Machine Learning Spring 2022
- What developers need to know about generative AI
Vector Database
- Vector database at AWS
- Vector database at Microsoft Semantic Kernel
- Vector database at Pinecone
- Vector database at Datastax
- Datastax Astra DB
- LanceDB
- How to get started with Milvus, via PyMilvus
- Vector Search Demos
Conversational Memory
- Conversational Memory with LangChain
- How to customize conversational memory
- Conversational Memory for LLMs with Langchain
Others
- Beginner’s Guide to FastAPI & OpenAI ChatGPT Integration
- Cube x LangChain: Building AI experiences with LLMs and the semantic layer
- LangChain + Chroma
- Introducing the Vercel AI SDK
Generative AI Terms Glossary
- Coursera Generative AI Definitions: A to Z Glossary Terms
- Product Hunt The ultimate A-Z guide to generative AI terminology
- C3.ai Generative AI Terms and Their Definitions
- Gartner Experts Answer the Top Generative AI Questions for Your Enterprise
- TechTarget - What is generative AI? Everything you need to know
- Scribbr - Glossary of AI Terms - Acronyms & Terminology
Recommended Books
- Generative Deep Learning: Teaching Machines to Paint, Write, Compose, and Play
- Modern Generative AI with ChatGPT and OpenAI Models: Leverage the capabilities of OpenAI’s LLM for productivity and innovation with GPT3 and GPT4
- Natural Language Processing with Transformers: Building Language Applications with Hugging Face
- LangChain AI Handbook
- 8 Best Generative AI Books of All Time by BookAuthority
- Top 8+ AI Prompt Engineering Books for 2023 – Learn Generative AI Prompts
- Top 6+ GAN Books – Generative Adversarial Networks And Generative AI
- The Master Algorithm
- Mathematics for Machine Learning
- Generative Image Dynamics
If you are interested in Citizen Development, refer to this book outline here on Empower Innovation: A Guide to Citizen Development in Microsoft 365
Now, available on | ||||
---|---|---|---|---|
![]() |
||||
![]() |
||||
![]() |
![]() |
![]() |
![]() |
![]() |
Also, you can look at this blog post series from various sources.
Stay tuned! on Generative AI Blog Series
We are advocating citizen development everywhere and empowering business users (budding citizen developers) to build their own solutions without software development experience, dogfooding cutting-edge technology, experimenting, crawling, falling, failing, restarting, learning, mastering, sharing, and becoming self-sufficient.
Please feel free to Book Time @ topmate! with our experts to get help with your Citizen Development adoption.
Comments